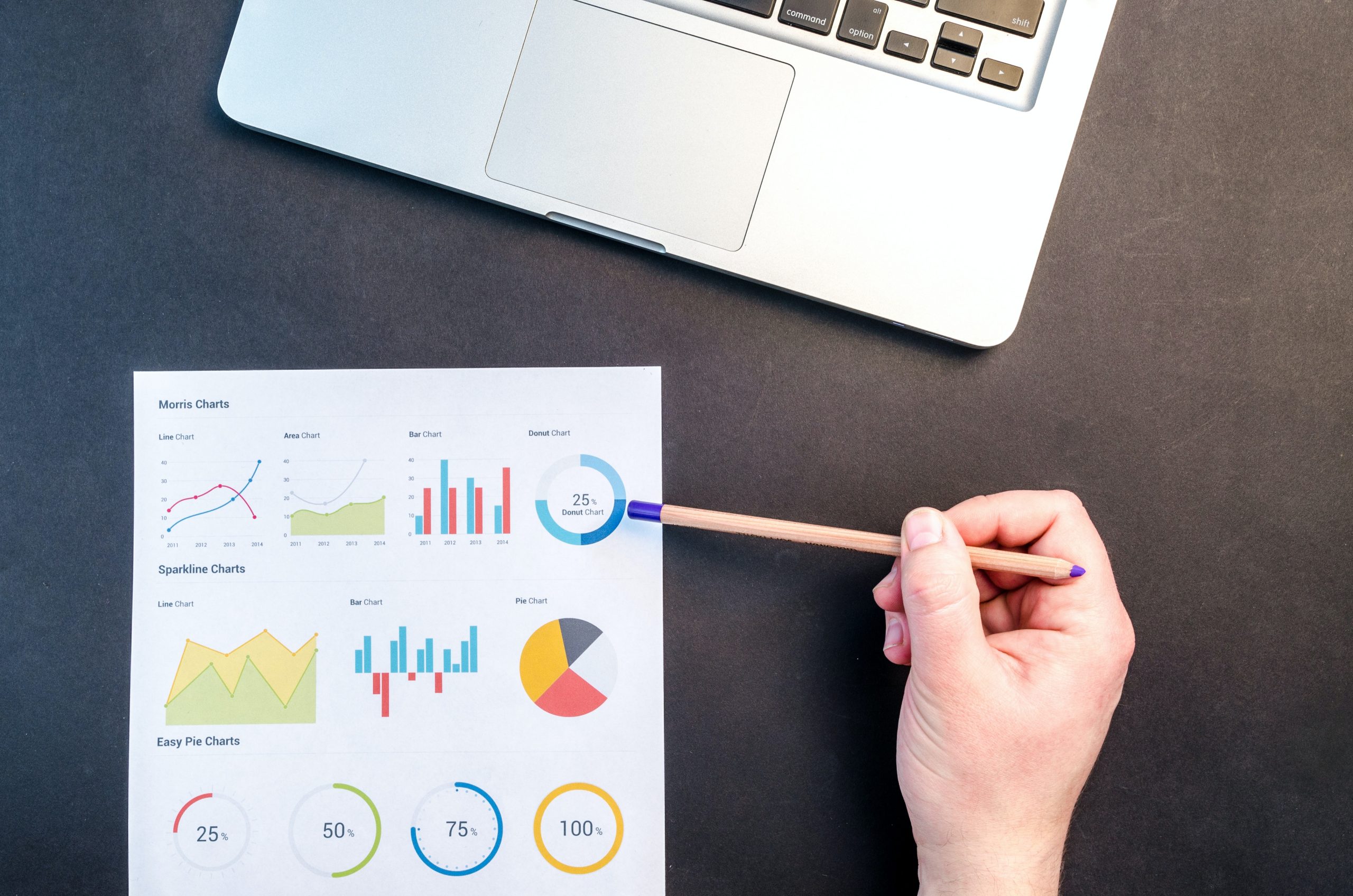
Standardized tests are widely used to determine student performance at all levels of education. However, a rater’s subjective assessment of the answers may affect the student’s score, particularly when examining open-ended or essay questions that are particularly valuable for providing insights into a student’s ability.
“A critical concern in rater-mediated performance assessments is how to evaluate and improve the quality of rater judgments,” said Jue Wang, assistant professor in the Department of Educational and Psychological Studies’ Research, Measurement & Evaluation Program. “Enhancing rating quality would make the system more valid, reliable, and fair in education and throughout society.”
Many studies have found that human raters introduce random and systematic biases into scoring decisions, such as different scoring based on gender, race or ethnicity. To address that concern, Wang is launching a new project, “Psychometric Modeling and the Evaluation of Rater Effects in Performance Assessments,” which was selected to receive a Provost’s Research Award for FY2022. Her work will involve developing a self-paced computerized adaptive testing (CAT) procedure for evaluating rater scoring proficiency.
Wang’s research will facilitate the creation of a training program that will provide tailored feedback to individual raters and thus improve the effectiveness and efficiency of rater training in performance assessments. “Most of the analyses in this project will be simulation based, and draw on datasets collected for prior analyses,” she said.
This project could have a significant impact on high-stakes assessments, such as Advanced Placement (AP) tests, Graduate Record Examinations (GRE), and statewide assessments in K-12 settings. Wang added that adaptive rater training program will be beneficial to various stakeholders, including academic institutions, raters, test-takers, and test administrators. It may also improve the use of automated scoring engines, which require human scoring for developing machine learning algorithms.
“The outbreak of COVID-19 has only exacerbated the problems in rater training due to an increasing demand for remote testing,” said Wang. “In the current situation, performance-based tasks with open-ended questions are less affected than multiple-choice questions by remote testing where test security issues may complicate the score inferences. However, it is also more challenging to deliver rater training and monitor rater scoring processes in the remote settings based on current training practices.
Wang’s work has been published in leading journals related to measurement, and she recently co-authored a book, “Rasch Models for Solving Measurement Problems: Invariant Measurement in the Social Sciences.”
As for her new project, Wang hopes it will promote a fairer education system with benefits throughout society. As she said, “By integrating CAT into the rater training program, we will improve the effectiveness and efficiency of training practices, ultimately enhancing the validity, reliability, and fairness of scoring decisions in the education system.”