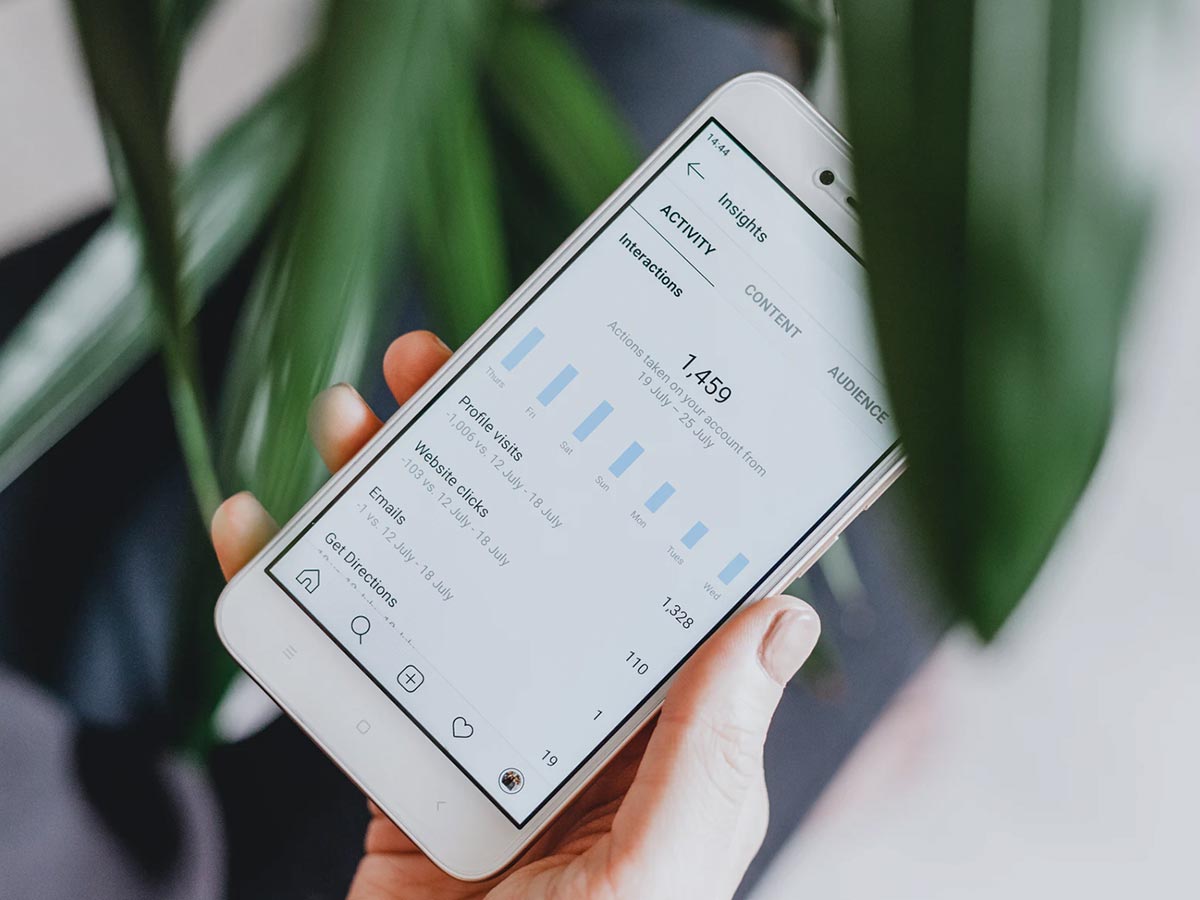
Biases in data collection and analysis can affect research results, particularly in fields like public health, education and social justice, according to Jennifer Kahn, assistant professor, Department of Teaching and Learning.
“The COVID-19 pandemic has disproportionately impacted Black and Hispanic communities as well as women who have had to leave the workforce to care for families,” said Kahn. “This far-reaching public health and economic crisis has highlighted the urgency for interventions that can counter biases in the data lifecycle to foster health, well-being, and fairness for community members and professionals.”
To advance that goal, Kahn is leading a collaborative initiative, “Project Data Inclusion,” supported by a 2020-2021 U-LINK Social Equity Rapid Response Award. She leads a multidisciplinary team of investigators, including Soyeon Ahn, professor and chair, Department of Education and Psychological Studies; Debbiesiu Lee, EPS associate professor; Ching-Hua Chuan, research associate professor of Interactive Media in the School of Communications; and Patricia Jones, assistant professor of medicine at the Miller School of Medicine.
Kahn and her team are currently developing an anti-bias training program in order to raise awareness of systemic inequities, and particularly their impacts on communities of color and women, across the data lifecycle—from data collection, analysis, modeling, storytelling, to decision-making processes.
This training, which will be piloted in the UM community this summer, follows a train-the-trainer design. Initial participants will be drawn from graduate students and faculty in public health, as well as psychological and educational fields. “They will become ambassadors to educate and engage in anti-bias efforts in their respective professional data contexts,” said Kahn, adding the initiative will also bring participants into conversation with local community stakeholders.
The first step in addressing data bias is awareness, according to Kahn. Next, students, educators and other professionals should look at how data has been collected to see if potential groups have been left out. Other issues to consider are understanding who has financed a study, as well as other stakeholders who might have a conscious or unconscious discriminatory bias. “Including community voices in the analysis and story-telling process can go a long way toward mitigating those adverse effects,” she said.
Kahn’s research has focused on how individuals learn to develop critical data literacy, particularly with unwieldy, complex large-scale datasets, to interpret and tell evidence-based, personally meaningful data stories.
“Along with training the trainers this summer, we plan to provide online resources, and develop a textbook for courses at the University of Miami that could serve as a national model,” Kahn said. “Students entering the fields of medicine, education and data sciences need to be aware of potential biases and other ethical concerns right at the start of their careers. It’s too important a topic to be considered an add-on at the end of their training.”